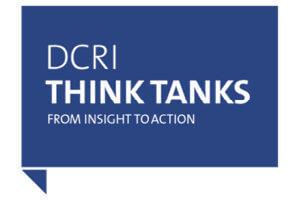
Stakeholders at a January 2020 DCRI Think Tank examined the role of machine learning (ML) in the design, conduct, and analysis of clinical trials. Key areas of clinical trial methodology were identified where ML holds particular promise and priority areas for further investigation.
Machine learning (ML) has potential to help improve the success, generalizability, patient-centeredness, and efficiency of clinical trials, write the authors of a paper recently published in in Trials. The paper concludes that ML holds great promise for improving the efficiency and quality of clinical research, but that significant barriers remain. To overcome these, evidence gaps—including the need for additional peer-reviewed investigations of the applications of ML to research—will need to be addressed.
The paper shares takeaways from a 2020 DCRI Think Tank entitled “Leveraging Artificial Intelligence and Machine Learning Methods and Approaches to Transform Clinical Trial Design, Planning, and Execution.” The lead author is DCRI’s E. Hope Weissler, MD; Duke Forge’s Erich Huang, PhD, MD (now Chief Science & Innovation Officer at Onduo, by Verily), was a co-director of this meeting and a co-author of the paper.
Participants examined ML roles in various areas of clinical research, including the pre-trial phase, cohort selection and participant management, and data collection and analysis. There was particular focus on operational and philosophical barriers to the use of ML in clinical research.
Think Tank participants included biomedical and ML researchers, representatives from the U.S. Food and Drug Administration (FDA), artificial intelligence technology and data analytics companies, nonprofit organizations, patient advocacy groups, and pharmaceutical companies.
Participants agreed that:
- Traditional double-blinded, randomized, controlled clinicals remain the gold standard for biomedical evidence generation.
- Augmentation with ML—from preclinical drug discovery to pre-trial planning through study execution to data management and analysis—may improve the success and efficiency of clinical research; if so, there is an ethical imperative to explore this possibility.
- To realize the potential of ML, solutions will need to be found for issues involving data structure, interoperability, and access, definitions of outcomes, transparency of development and validation processes, objectivity of certification, and the possibility of bias.
- Communication and collaboration will be needed to realize benefits from ML in clinical evidence generation. Transparent discussion about potential benefits and drawbacks and the sharing of best practices must continue. ML will only improve the quality and efficiency of research if it is applied in a fair, ethical, and open manner.
Additional DCRI authors of the paper were Lesley Curtis, PhD, former DCRI faculty member Scott Kollins, PhD, and former DCRI faculty member Matthew Roe, MD, MHS.